The Three Pillars for Reliable Research
When working in quantitative research, data integrity is essential. When the validity of numbers is compromised, market research loses its value. Amidst the benefits brought by AI, automation, and global panel marketplaces, challenges like bots and panel fraud have surfaced, necessitating robust data integrity practices. This article delves into the three pillars essential for achieving true data integrity: the right people, the right questions, and the right data.
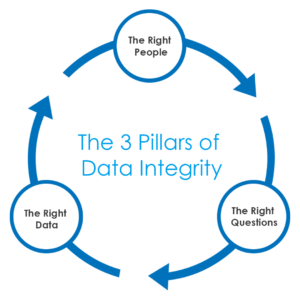
Pillar 1: The Right People
The foundation of reliable data lies in recruiting genuine and thoughtful participants. Ensuring participants are real, attentive, reliable, and applicable to the study is critical. There are two primary methods to achieve this. The first involves developing an internal panel, which allows for direct engagement and ongoing verification of participants. By building trust over time, researchers can rely on participants who have previously demonstrated high-quality engagement. This approach is also cost-effective, reducing the need for external panels.
The second method is partnering with trusted panels. Collaborating with recommended suppliers who meet ISO quality standards ensures the legitimacy of participants. Understanding their participant verification methods is crucial to maintaining data quality.
Additionally, improving the participant experience can help retain quality participants and reduce dropout rates. To retain quality participants and reduce dropout rates:
- Clear and concise screeners: Avoid complex or confusing questions that may lead to participant frustration.
- Minimise industry jargon: Use language familiar to the general public to prevent misunderstandings.
- Manage participant relationships: Provide guidance and clear information at every stage.
Pillar 2: The Right Questions
Managing participant fatigue is essential to maintaining data quality. There are three types of fatigue to watch out for:
- Inherent fatigue: Participants who were never truly engaged from the start.
- Break fatigue: Participants who encounter a challenging or lengthy question and decide to quit.
- Accumulated fatigue: Participants who gradually lose interest as the survey progresses.
To avoid inherent fatigue, it is essential to design engaging and relevant screeners that filter out those who are not genuinely interested in the study. This can be achieved by making the initial questions interesting and ensuring they relate closely to the participants’ experiences or interests. Additionally, detailed data cleaning can help identify participants displaying inherent fatigue through poor open-ended responses or repetitive answers, allowing researchers to filter out low-quality data early on.
As stated, break fatigue occurs when participants encounter a challenging or lengthy question and decide to quit. To prevent this, researchers should avoid placing complex or lengthy questions in the middle of surveys. Instead, breaking down large grids into smaller, more manageable sections and using varied question types can keep participants engaged. Additionally, placing difficult questions towards the end of the survey can help retain participants’ interest throughout.
Accumulated fatigue can be mitigated by designing shorter, more focused surveys. Ensuring that each question is essential and avoiding repetition can help maintain participant engagement. Regularly changing the format of questions and incorporating interactive elements can also help keep the survey experience fresh and engaging.
Pillar 3: The Right Data
Effective data cleaning involves multiple checks to ensure accuracy and reliability. Verifying participant authenticity through IP addresses, metadata, and direct validation confirms their identities. Detecting and removing fraudulent responses involves identifying patterns that suggest bots or repeat entries. Analysing the quality of open-ended responses helps detect gibberish or AI-generated text.
AI and automation can significantly aid in data cleaning, but they should complement rather than replace manual oversight. AI tools excel at identifying patterns across large datasets, making them useful for initial cleaning checks to flag obvious issues. They can also analyse complex data relationships and draw connections between participant responses.
Conclusion
Maintaining data integrity requires a comprehensive approach encompassing careful participant selection, thoughtful question design, and rigorous data cleaning. By adhering to these three pillars, researchers can ensure the reliability and validity of their data, ultimately enhancing the value of their research outcomes. In an era where data integrity faces new challenges, these best practices provide a robust framework for delivering trustworthy insights.
If you have any questions or if you’d like to discuss anything further, please reach out to [email protected]
Stay In Touch